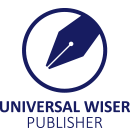
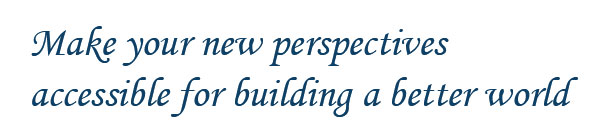
|
|
||||
|
Deadline for Submissions: 30 August 2025 |
||||
|
Special Issue Editors |
||||
|
|
||||
|
Guest Editor |
Prof. Gerhard-Wilhelm Weber |
|
|
|
|
Affiliation |
Faculty of Engineering Management, Poznan University of Technology, Poland |
|||
|
|
||||
|
Guest Editor |
Dr. Alireza Goli |
|
|
|
|
Affiliation |
Department of Industrial Engineering, University of Isfahan, Isfahan, Iran |
|||
|
|
||||
|
Guest Editor |
Prof. Mika Sillanpää |
|
|
|
|
Affiliation |
Department of Chemical Engineering, School of Mining, Metallurgy and Chemical Engineering, University of Johannesburg, P. O. Box 17011, Doornfontein, 2028, South Africa |
|||
|
|
||||
|
Guest Editor |
Dr. Toufik Mzili |
|
|
|
|
Affiliation |
Department of Computer Science, Chouaib Doukkali University, El Jadida, Morocco |
|||
|
|
|
|
||
|
|
||||
|
Special Issue Information |
||||
|
In the age of big data and complex systems, data-driven optimization has become essential for decision-making processes across numerous fields, from industrial engineering to healthcare, finance, and environmental management. However, real-world data often contain high levels of uncertainty and imprecision due to factors such as measurement errors, incomplete data, or unpredictable changes in conditions. Incorporating uncertainty and fuzziness within data-driven optimization frameworks has therefore become crucial for developing robust, adaptable solutions that can perform well under diverse, uncertain conditions. This Special Issue seeks to explore cutting-edge approaches for integrating uncertainty and fuzziness into data-driven optimization models, enhancing the reliability and resilience of decision-making systems. By leveraging techniques such as fuzzy set theory, probabilistic modeling, stochastic optimization, and hybrid methods, researchers can create algorithms that account for both structured and unstructured uncertainty, ultimately improving system performance and interpretability. Furthermore, combining these methods with machine learning and artificial intelligence (AI) enables the development of adaptive, self-correcting models that can dynamically respond to evolving data patterns. A key focus of this issue is to highlight innovative applications of uncertainty and fuzziness in real-world optimization scenarios. Example applications include supply chain management, resource allocation, predictive maintenance, and risk assessment, where the ability to handle imprecise or ambiguous data can make a significant difference in outcomes. Researchers are encouraged to contribute both theoretical advancements and applied case studies that illustrate the effectiveness of these techniques in practical settings, showcasing how they can enhance the adaptability and robustness of decision-making frameworks. We invite researchers, practitioners, and experts to submit original research articles, comprehensive reviews, and insightful case studies on topics that include fuzzy optimization, stochastic processes, robust optimization, interval analysis, and AI-driven approaches for uncertainty management. By compiling these works, this Special Issue aims to provide a comprehensive view of the latest advancements in integrating uncertainty and fuzziness into data-driven optimization, promoting cross-disciplinary collaboration and innovation in this growing field. We invite researchers and practitioners to submit original research and critical survey manuscripts that propose novel approach on the following potential topics and their applications, but are not limited to: Hybrid Fuzzy and Data-Driven Optimization Models for Uncertain Environments Application of Fuzzy Systems and Probabilistic Methods Data-Driven Fuzzy Optimization for Robustness in Complex Systems Incorporating Nonstandard Logic Systems Aggregation Techniques for Decision-Making and Risk Assessment Leveraging Machine Learning and Evolutionary Computation Fuzzy Optimization Techniques for Real-Time Resource Allocation Advanced Data Analytics and Data Science Applications Renewable Energy Optimization through Evidence-Based Mathematical Approaches Circular Economy and Sustainable Supply Chain Design Fuzzy and Probabilistic Methods in Stochastic Optimization Fuzzy-Neural Networks and Data-Driven Models for Complex Decision-Making
|
||||
|
|
||||
|
Published Papers |
||||
|
|
||||
|
This special issue is now open for submission. |
||||
|
|